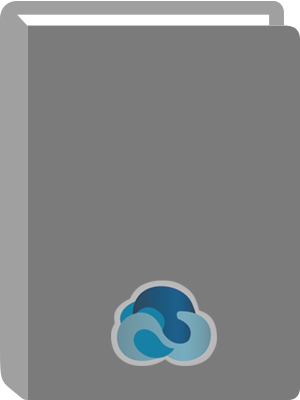
Measuring Scholarly Impact Methods and Practice
Başlık:
Measuring Scholarly Impact Methods and Practice
Yazar:
Ding, Ying. editor.
ISBN:
9783319103778
Fiziksel Niteleme:
XIV, 346 p. 89 illus., 68 illus. in color. online resource.
İçindekiler:
Community detection and visualization of networks with the map equation framework -- Link Prediction -- Network analysis and indicators -- PageRank-related methods for analyzing citation networks -- Systems Life Cycle and its relation with the Triple Helix -- Spatial scientometrics and scholarly impact: A review of recent studies, tools and methods -- Researchers’ publication patterns and their use for author disambiguation -- Knowledge integration and diffusion: Measures and mapping of diversity and coherence -- Limited dependent variables models and probabilistic prediction in informetrics -- Text mining with the Stanford CoreNLP -- Topic modeling: Measuring scholarly impact using a topical lens -- The substantive and practical significance of citation impact differences between institutions: Guidelines for the analysis of percentiles using effect sizes and confidence intervals -- Visualizing bibliometric networks -- Replicable science of science studies.
Özet:
This book is an authoritative handbook of current topics, technologies and methodological approaches that may be used for the study of scholarly impact. The included methods cover a range of fields such as statistical sciences, scientific visualization, network analysis, text mining, and information retrieval. The techniques and tools enable researchers to investigate metric phenomena and to assess scholarly impact in new ways. Each chapter offers an introduction to the selected topic and outlines how the topic, technology or methodological approach may be applied to metrics-related research. Comprehensive and up-to-date, Measuring Scholarly Impact: Methods and Practice is designed for researchers and scholars interested in informetrics, scientometrics, and text mining. The hands-on perspective is also beneficial to advanced-level students in fields from computer science and statistics to information science.
Ek Kurum Yazar:
Elektronik Erişim:
http://dx.doi.org/10.1007/978-3-319-10377-8