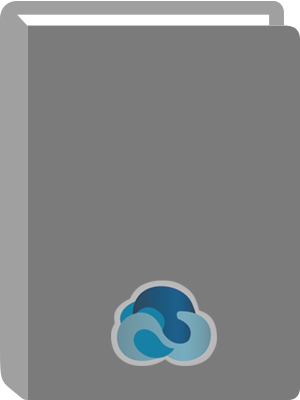
Reconstruction, Identification and Implementation Methods for Spiking Neural Circuits
Başlık:
Reconstruction, Identification and Implementation Methods for Spiking Neural Circuits
Yazar:
Florescu, Dorian. author.
ISBN:
9783319570815
Yazar:
Edisyon:
1st ed. 2017.
Fiziksel Niteleme:
XIV, 139 p. 42 illus., 27 illus. in color. online resource.
Seri:
Springer Theses, Recognizing Outstanding Ph.D. Research,
İçindekiler:
Nomenclature -- Acronyms -- 1 Introduction -- 2 Time Encoding and Decoding in Bandlimited and Shift-Invariant Spaces -- 3 A Novel Framework for Reconstructing Bandlimited Signals Encoded by Integrate and-Fire Neurons -- 4 A Novel Reconstruction Framework in Shift-Invariant Spaces for Signals Encoded with Integrate-and-Fire Neurons -- 5 A New Approach to the Identification of Sensory Processing Circuits Based on Spiking Neuron Data -- 6 A New Method for Implementing Linear Filters in the Spike Domain -- 7 Conclusions and Future Work -- Bibliography.
Özet:
This work is motivated by the ongoing open question of how information in the outside world is represented and processed by the brain. Consequently, several novel methods are developed. A new mathematical formulation is proposed for the encoding and decoding of analog signals using integrate-and-fire neuron models. Based on this formulation, a novel algorithm, significantly faster than the state-of-the-art method, is proposed for reconstructing the input of the neuron. Two new identification methods are proposed for neural circuits comprising a filter in series with a spiking neuron model. These methods reduce the number of assumptions made by the state-of-the-art identification framework, allowing for a wider range of models of sensory processing circuits to be inferred directly from input-output observations. A third contribution is an algorithm that computes the spike time sequence generated by an integrate-and-fire neuron model in response to the output of a linear filter, given the input of the filter encoded with the same neuron model.
Konu Başlığı:
Ek Kurum Yazar:
Elektronik Erişim:
https://doi.org/10.1007/978-3-319-57081-5