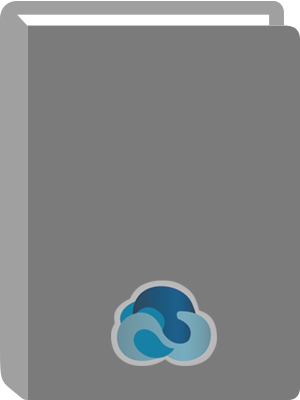
Intravascular Imaging and Computer Assisted Stenting, and Large-Scale Annotation of Biomedical Data and Expert Label Synthesis 6th Joint International Workshops, CVII-STENT 2017 and Second International Workshop, LABELS 2017, Held in Conjunction with MICCAI 2017, Québec City, QC, Canada, September 10–14, 2017, Proceedings
Başlık:
Intravascular Imaging and Computer Assisted Stenting, and Large-Scale Annotation of Biomedical Data and Expert Label Synthesis 6th Joint International Workshops, CVII-STENT 2017 and Second International Workshop, LABELS 2017, Held in Conjunction with MICCAI 2017, Québec City, QC, Canada, September 10–14, 2017, Proceedings
Yazar:
Cardoso, M. Jorge. editor.
ISBN:
9783319675343
Edisyon:
1st ed. 2017.
Fiziksel Niteleme:
XVI, 166 p. 73 illus. online resource.
Seri:
Image Processing, Computer Vision, Pattern Recognition, and Graphics ; 10552
Özet:
This book constitutes the refereed joint proceedings of the 6th Joint International Workshop on Computing and Visualization for Intravascular Imaging and Computer Assisted Stenting, CVII-STENT 2017, and the Second International Workshop on Large-Scale Annotation of Biomedical Data and Expert Label Synthesis, LABELS 2017, held in conjunction with the 20th International Conference on Medical Imaging and Computer-Assisted Intervention, MICCAI 2017, in Québec City, QC, Canada, in September 2017. The 6 full papers presented at CVII-STENT 2017 and the 11 full papers presented at LABELS 2017 were carefully reviewed and selected. The CVII-STENT papers feature the state of the art in imaging, treatment, and computer-assisted intervention in the field of endovascular interventions. The LABELS papers present a variety of approaches for dealing with few labels, from transfer learning to crowdsourcing.
Konu Başlığı:
Ek Kurum Yazar:
Elektronik Erişim:
https://doi.org/10.1007/978-3-319-67534-3