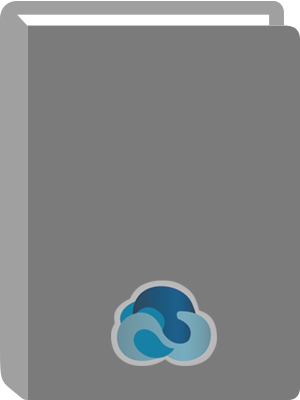
An architecture for fast and general data processing on large clusters
Başlık:
An architecture for fast and general data processing on large clusters
Yazar:
Zaharia, Matei., author.
ISBN:
9781970001570
Yazar:
Edisyon:
First edition.
Fiziksel Niteleme:
1 PDF (xii, 128 pages) : illustrations.
Seri:
ACM books, #11
ACM books ; #11.
İçindekiler:
1. Introduction -- 1.1 Problems with specialized systems -- 1.2 Resilient distributed datasets (RDDs) -- 1.3 Models implemented over RDDs -- 1.4 Summary of results -- 1.5 Book overview --
2. Resilient distributed datasets -- 2.1 Introduction -- 2.2 RDD abstraction -- 2.3 Spark programming interface -- 2.4 Representing RDDs -- 2.5 Implementation -- 2.6 Evaluation -- 2.7 Discussion -- 2.8 Related work -- 2.9 Summary --
3. Models built over RDDs -- 3.1 Introduction -- 3.2 Techniques for implementing other models on RDDs -- 3.3 Shark: SQL on RDDs -- 3.4 Implementation -- 3.5 Performance -- 3.6 Combining SQL with complex analytics -- 3.7 Summary --
4. Discretized streams -- 4.1 Introduction -- 4.2 Goals and background -- 4.3 Discretized streams (D-streams) -- 4.4 System architecture -- 4.5 Fault and straggler recovery -- 4.6 Evaluation -- 4.7 Discussion -- 4.8 Related work -- 4.9 Summary --
5. Generality of RDDs -- 5.1 Introduction -- 5.2 Expressiveness perspective -- 5.3 Systems perspective -- 5.4 Limitations and extensions -- 5.5 Related work -- 5.6 Summary --
6. Conclusion -- 6.1 Lessons learned -- 6.2 Evolution of spark in industry -- 6.3 Future work -- References -- Author's biography.
Özet:
The past few years have seen a major change in computing systems, as growing data volumes and stalling processor speeds require more and more applications to scale out to clusters. Today, a myriad data sources, from the Internet to business operations to scientific instruments, produce large and valuable data streams. However, the processing capabilities of single machines have not kept up with the size of data. As a result, organizations increasingly need to scale out their computations over clusters. At the same time, the speed and sophistication required of data processing have grown. In addition to simple queries, complex algorithms like machine learning and graph analysis are becoming common. And in addition to batch processing, streaming analysis of real-time data is required to let organizations take timely action. Future computing platforms will need to not only scale out traditional workloads, but support these new applications too.
Elektronik Erişim:
Abstract with links to full text http://dx.doi.org/10.1145/2886107