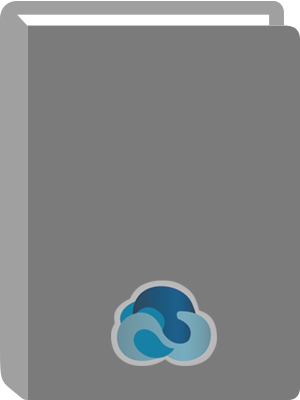
Models and Algorithms for Biomolecules and Molecular Networks.
Başlık:
Models and Algorithms for Biomolecules and Molecular Networks.
Yazar:
Bhaskar DasGupta, author.
ISBN:
9781119162254
Yazar:
Fiziksel Niteleme:
1 PDF (263).
Seri:
IEEE Press Series on Biomedical Engineering 30
IEEE Press Series on Biomedical Engineering ; 30
İçindekiler:
List of Figures xiii -- List of Tables xix -- Foreword xxi -- Acknowledgments xxiii -- 1 Geometric Models of Protein Structure and Function Prediction 1 -- 1.1 Introduction, 1 -- 1.2 Theory and Model, 2 -- 1.2.1 Idealized Ball Model, 2 -- 1.2.2 Surface Models of Proteins, 3 -- 1.2.3 Geometric Constructs, 4 -- 1.2.4 Topological Structures, 6 -- 1.2.5 Metric Measurements, 9 -- 1.3 Algorithm and Computation, 13 -- 1.4 Applications, 15 -- 1.4.1 Protein Packing, 15 -- 1.4.2 Predicting Protein Functions from Structures, 17 -- 1.5 Discussion and Summary, 20 -- References, 22 -- Exercises, 25 -- 2 Scoring Functions for Predicting Structure and Binding of Proteins 29 -- 2.1 Introduction, 29 -- 2.2 General Framework of Scoring Function and Potential Function, 31 -- 2.2.1 Protein Representation and Descriptors, 31 -- 2.2.2 Functional Form, 32 -- 2.2.3 Deriving Parameters of Potential Functions, 32 -- 2.3 Statistical Method, 32 -- 2.3.1 Background, 32 -- 2.3.2 Theoretical Model, 33 -- 2.3.3 Miyazawa -- Jernigan Contact Potential, 34 -- 2.3.4 Distance-Dependent Potential Function, 41 -- 2.3.5 Geometric Potential Functions, 45 -- 2.4 Optimization Method, 49 -- 2.4.1 Geometric Nature of Discrimination, 50 -- 2.4.2 Optimal Linear Potential Function, 52 -- 2.4.3 Optimal Nonlinear Potential Function, 53 -- 2.4.4 Deriving Optimal Nonlinear Scoring Function, 55 -- 2.4.5 Optimization Techniques, 55 -- 2.5 Applications, 55 -- 2.5.1 Protein Structure Prediction, 56 -- 2.5.2 Protein -- Protein Docking Prediction, 56 -- 2.5.3 Protein Design, 58 -- 2.5.4 Protein Stability and Binding Affinity, 59 -- 2.6 Discussion and Summary, 60 -- 2.6.1 Knowledge-Based Statistical Potential Functions, 60 -- 2.6.2 Relationship of Knowledge-Based Energy Functions and Further Development, 64 -- 2.6.3 Optimized Potential Function, 65 -- 2.6.4 Data Dependency of Knowledge-Based Potentials, 66 -- References, 67 -- Exercises, 75 -- 3 Sampling Techniques: Estimating Evolutionary Rates and Generating Molecular Structures 79.
3.1 Introduction, 79 -- 3.2 Principles of Monte Carlo Sampling, 81 -- 3.2.1 Estimation Through Sampling from Target Distribution, 81 -- 3.2.2 Rejection Sampling, 82 -- 3.3 Markov Chains and Metropolis Monte Carlo Sampling, 83 -- 3.3.1 Properties of Markov Chains, 83 -- 3.3.2 Markov Chain Monte Carlo Sampling, 85 -- 3.4 Sequential Monte Carlo Sampling, 87 -- 3.4.1 Importance Sampling, 87 -- 3.4.2 Sequential Importance Sampling, 87 -- 3.4.3 Resampling, 91 -- 3.5 Applications, 92 -- 3.5.1 Markov Chain Monte Carlo for Evolutionary Rate Estimation, 92 -- 3.5.2 Sequentail Chain Growth Monte Carlo for Estimating Conformational Entropy of RNA Loops, 95 -- 3.6 Discussion and Summary, 96 -- References, 97 -- Exercises, 99 -- 4 Stochastic Molecular Networks 103 -- 4.1 Introduction, 103 -- 4.2 Reaction System and Discrete Chemical Master Equation, 104 -- 4.3 Direct Solution of Chemical Master Equation, 106 -- 4.3.1 State Enumeration with Finite Buffer, 106 -- 4.3.2 Generalization and Multi-Buffer dCME Method, 108 -- 4.3.3 Calculation of Steady-State Probability Landscape, 108 -- 4.3.4 Calculation of Dynamically Evolving Probability Landscape, 108 -- 4.3.5 Methods for State Space Truncation for Simplification, 109 -- 4.4 Quantifying and Controlling Errors from State Space Truncation, 111 -- 4.5 Approximating Discrete Chemical Master Equation, 114 -- 4.5.1 Continuous Chemical Master Equation, 114 -- 4.5.2 Stochastic Differential Equation: Fokker -- Planck Approach, 114 -- 4.5.3 Stochastic Differential Equation: Langevin Approach, 116 -- 4.5.4 Other Approximations, 117 -- 4.6 Stochastic Simulation, 118 -- 4.6.1 Reaction Probability, 118 -- 4.6.2 Reaction Trajectory, 118 -- 4.6.3 Probability of Reaction Trajectory, 119 -- 4.6.4 Stochastic Simulation Algorithm, 119 -- 4.7 Applications, 121 -- 4.7.1 Probability Landscape of a Stochastic Toggle Switch, 121 -- 4.7.2 Epigenetic Decision Network of Cellular Fate in Phage Lambda, 123 -- 4.8 Discussions and Summary, 127 -- References, 128.
Exercises, 131 -- 5 Cellular Interaction Networks 135 -- 5.1 Basic Definitions and Graph-Theoretic Notions, 136 -- 5.1.1 Topological Representation, 136 -- 5.1.2 Dynamical Representation, 138 -- 5.1.3 Topological Representation of Dynamical Models, 139 -- 5.2 Boolean Interaction Networks, 139 -- 5.3 Signal Transduction Networks, 141 -- 5.3.1 Synthesizing Signal Transduction Networks, 142 -- 5.3.2 Collecting Data for Network Synthesis, 146 -- 5.3.3 Transitive Reduction and Pseudo-node Collapse, 147 -- 5.3.4 Redundancy and Degeneracy of Networks, 153 -- 5.3.5 Random InteractionNetworks and Statistical Evaluations, 157 -- 5.4 Reverse Engineering of Biological Networks, 159 -- 5.4.1 Modular Response Analysis Approach, 160 -- 5.4.2 Parsimonious Combinatorial Approaches, 166 -- 5.4.3 Evaluation of Quality of the Reconstructed Network, 171 -- References, 173 -- Exercises, 178 -- 6 Dynamical Systems and Interaction Networks 183 -- 6.1 Some Basic Control-Theoretic Concepts, 185 -- 6.2 Discrete-Time Boolean Network Models, 186 -- 6.3 Artificial Neural Network Models, 188 -- 6.3.1 Computational Powers of ANNs, 189 -- 6.3.2 Reverse Engineering of ANNs, 190 -- 6.3.3 Applications of ANN Models in Studying Biological Networks, 192 -- 6.4 Piecewise Linear Models, 192 -- 6.4.1 Dynamics of PL Models, 194 -- 6.4.2 Biological Application of PL Models, 195 -- 6.5 Monotone Systems, 200 -- 6.5.1 Definition of Monotonicity, 201 -- 6.5.2 Combinatorial Characterizations and Measure of Monotonicity, 203 -- 6.5.3 Algorithmic Issues in Computing the Degree of Monotonicity ��, 207 -- References, 209 -- Exercises, 214 -- 7 Case Study of Biological Models 217 -- 7.1 Segment Polarity Network Models, 217 -- 7.1.1 Boolean Network Model, 218 -- 7.1.2 Signal Transduction Network Model, 218 -- 7.2 ABA-Induced Stomatal Closure Network, 219 -- 7.3 Epidermal Growth Factor Receptor Signaling Network, 220 -- 7.4 C. elegans Metabolic Network, 223 -- 7.5 Network for T-Cell Survival and Death in Large Granular Lymphocyte Leukemia, 223.
References, 224 -- Exercises, 225 -- Glossary 227 -- Index 229.
Tür:
Elektronik Erişim:
Abstract with links to resource http://ieeexplore.ieee.org/xpl/bkabstractplus.jsp?bkn=7434880