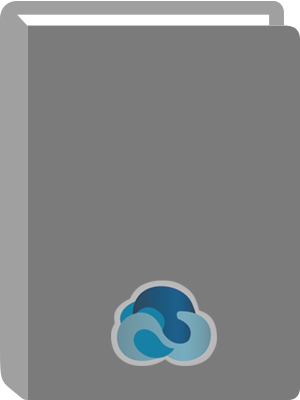
Machine Learning for Medical Image Reconstruction Second International Workshop, MLMIR 2019, Held in Conjunction with MICCAI 2019, Shenzhen, China, October 17, 2019, Proceedings
Başlık:
Machine Learning for Medical Image Reconstruction Second International Workshop, MLMIR 2019, Held in Conjunction with MICCAI 2019, Shenzhen, China, October 17, 2019, Proceedings
Yazar:
Knoll, Florian. editor. (orcid)0000-0001-5357-8656
ISBN:
9783030338435
Edisyon:
1st ed. 2019.
Fiziksel Niteleme:
IX, 266 p. 128 illus., 94 illus. in color. online resource.
Seri:
Image Processing, Computer Vision, Pattern Recognition, and Graphics ; 11905
İçindekiler:
Deep Learning for Magnetic Resonance Imaging -- Recon-GLGAN: A Global-Local context based Generative Adversarial Network for MRI Reconstruction- Self-supervised Recurrent Neural Network for 4D Abdominal and In-utero MR Imaging -- Fast Dynamic Perfusion and Angiography Reconstruction using an end-to-end 3D Convolutional Neural Network -- APIR-Net: Autocalibrated Parallel Imaging Reconstruction using a Neural Network -- Accelerated MRI Reconstruction with Dual-domain Generative Adversarial Network -- Deep Learning for Low-Field to High-Field MR: Image Quality Transfer with Probabilistic Decimation Simulator -- Joint Multi-Anatomy Training of a Variational Network for Reconstruction of Accelerated Magnetic Resonance Image Acquisitions -- Modeling and Analysis Brain Development via Discriminative Dictionary Learning -- Deep Learning for Computed Tomography -- Virtual Thin Slice: 3D Conditional GAN-based Super-resolution for CT Slice Interval -- Data Consistent Artifact Reduction for Limited Angle Tomography with Deep Learning Prior -- Measuring CT Reconstruction Quality with Deep Convolutional Neural Networks -- Deep Learning based Metal Inpainting in the Projection Domain: Initial Results -- Deep Learning for General Image Reconstruction -- Flexible Conditional Image Generation of Missing Data with Learned Mental Maps -- Spatiotemporal PET reconstruction using ML-EM with learned diffeomorphic deformation -- Stain Style Transfer using Transitive Adversarial Networks -- Blind Deconvolution Microscopy Using Cycle Consistent CNN with Explicit PSF Layer -- Deep Learning based approach to quantification of PET tracer uptake in small tumors -- Task-GAN: Improving Generative Adversarial Network for Image Reconstruction -- Gamma Source Location Learning from Synthetic Multi-Pinhole Collimator Data -- Neural Denoising of Ultra-Low Dose Mammography -- Image Reconstruction in a Manifold of Image Patches: Application to Whole-fetus Ultrasound Imaging -- Image Super Resolution via Bilinear Pooling: Application to Confocal Endomicroscopy -- TPSDicyc: Improved Deformation Invariant Cross-domain Medical Image Synthesis -- PredictUS: A Method to Extend the Resolution-Precision Trade-off in Quantitative Ultrasound Image Reconstruction.
Özet:
This book constitutes the refereed proceedings of the Second International Workshop on Machine Learning for Medical Reconstruction, MLMIR 2019, held in conjunction with MICCAI 2019, in Shenzhen, China, in October 2019. The 24 full papers presented were carefully reviewed and selected from 32 submissions. The papers are organized in the following topical sections: deep learning for magnetic resonance imaging; deep learning for computed tomography; and deep learning for general image reconstruction.
Konu Başlığı:
Ek Kurum Yazar:
Elektronik Erişim:
https://doi.org/10.1007/978-3-030-33843-5